Manufacturers face critical challenges that impact both their revenue and costs. Accurate demand forecasting directly affects sales and market opportunities, while unplanned equipment breakdowns and supply chain disruptions drive operational costs. These issues lead to production delays, unhappy customers, and missed business opportunities, making it harder to stay competitive. Traditional methods often fail to spot these hidden problems, leaving room for significant improvements across the business.
However, advancements in business analytics are changing the game. With real-time data insights and predictive capabilities, manufacturers can make informed decisions to address these challenges head-on. This shift has led to a surge in the global advanced analytics market, valued at USD 49.81 billion in 2023 and growing to USD 60.33 billion in 2024. The market is expected to reach around USD 338.71 billion by 2033, expanding at a solid CAGR of 21.13% from 2024 to 2033.

This article explores the role of business analytics in the manufacturing industry and how it can transform operations. We’ll dive into strategies that help reduce costs, boost efficiency, and anticipate market demands, offering insights you can use to stay ahead of the competition.
Read on to uncover practical use cases, implementation tips, and upcoming trends that could redefine how you approach manufacturing.
Overview of Business Analytics in Manufacturing
Data analytics in the manufacturing industry leverages data from machines, operations, and systems to optimize production. This includes key functions such as maintenance, quality control, and planning. Manufacturers can use accurate, real-time data to make faster, better decisions that enhance productivity and efficiency.
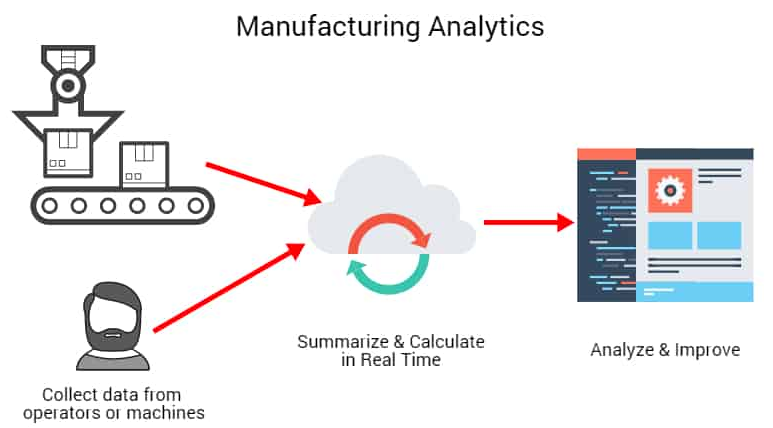
Traditional Challenges:
For years, manufacturers used data to improve efficiency and increase market share.
However, many still rely on outdated, manual data collection methods:
- Staff manually check and record machine performance, fill out forms, and maintain operation and maintenance logs.
- These methods are prone to human errors, introduce bias, and consume valuable time.
- The data gathered this way needs more depth and accuracy for precise analysis and decision-making.
Digital Transformation:
- The shift to digital data collection has revolutionized the industry.
- Connected devices and IoT-enabled machines now automate much of the data collection process.
- This shift reduces labor costs and delivers real-time, actionable insights through advanced analytics and algorithms.
Impact of Automation:
Automated machine data collection enables advanced use cases, such as:
- Predictive Maintenance: Anticipating equipment failures before they occur.
- Process Automation: Streamlining workflows for improved efficiency.
- Real-Time Monitoring: Tracking performance and identifying trends as they develop.
Key Benefits of Business Analytics in Manufacturing
Business analytics transforms manufacturers' operations, enabling data-driven decisions that boost productivity and cut costs. This shift is particularly evident in the North American market, where the advanced analytics sector is expanding rapidly. Valued at USD 22.91 billion in 2023, the market is projected to reach approximately USD 157.50 billion by 2033, growing at a robust CAGR of 21.26% from 2024 to 2033.
Here are the key benefits that manufacturers gain through the strategic use of analytics:
1. Cost Optimization
Every manufacturer prioritizes cost optimization, and business analytics provides the tools to achieve this. Manufacturers can identify inefficiencies and streamline operations by analyzing data from various production processes.
Here’s how analytics contributes to cost savings:
- Identifying Waste: This process pinpoints areas of overuse in materials, energy, and time, helping to reduce unnecessary expenses.
- Predictive Maintenance: Uses real-time equipment data to anticipate breakdowns, allowing maintenance to be performed before a failure occurs.some text
- Reduces unplanned downtime and associated repair costs.
- Extends the lifespan of machinery through timely intervention.
- Resource Allocation: Optimizes energy and material usage, lowering overall operational costs while maintaining productivity.
2. Increased Sales
Business analytics streamlines operations and directly contributes to boosting manufacturers' sales. By providing deeper insights into customer preferences, market trends, and purchasing behaviors, analytics helps manufacturers tailor their offerings to meet demand more effectively.
Here's how it impacts sales:
- Personalized Product Offerings: Analytics helps identify what customers seek, allowing manufacturers to adjust products and services to align better with market demand.
- Targeted Marketing Strategies: Uses data to craft more effective marketing campaigns, ensuring that the right products reach the right audience at the right time.
- Faster Response to Market Trends: Real-time insights enable manufacturers to react quickly to shifts in customer demand, ensuring that they capitalize on new opportunities before competitors.
- Optimized Pricing Models: Analyzing sales data helps set competitive prices that attract customers while maintaining healthy profit margins, ultimately driving more sales.
3. Enhanced Efficiency
Efficiency is a game-changer in manufacturing, and analytics helps achieve it by highlighting opportunities for improvement:
- Bottleneck Detection: Identifies slowdowns in production lines, enabling targeted improvements.
- Automation of Manual Tasks: Spotting repetitive processes that slow down your team and turning them into quick, automated workflows.
- Real-Time Adjustments: Allows adjustments to production schedules and resource use on the fly, leading to:
- Better equipment utilization.
- Reduced energy consumption and waste.
- Steady production flow, minimizing delays and excess inventory.
4. Quality Control
Maintaining high-quality standards is crucial for customer satisfaction and brand reputation. Analytics aids in achieving consistent quality throughout the manufacturing process:
- Immediate Quality Checks: Real-time sensor data allows for early detection of defects during production.
- Cost Savings: Reduces the need for rework and minimizes costs associated with defective products.
- Customer Satisfaction: Consistent product quality leads to fewer returns and higher customer trust.
- Risk Reduction: Lowers the chance of product recalls by maintaining high standards throughout the production cycle.
5. Supply Chain Optimization
An optimized supply chain ensures manufacturers can meet demand without incurring unnecessary costs. Business analytics provides critical insights for supply chain management:
- End-to-End Visibility: Offers a clear view of inventory levels, supplier performance, and logistics.
- Accurate Demand Forecasting: Predicts demand changes, enabling better stock management.
- Leverages sales data and market trends for accurate demand forecasting
- Optimizes inventory management to prevent stockouts and overstocking
- Adjusts production schedules dynamically to minimize waste and maximize resources
- Streamlined Logistics: Enhances coordination with suppliers and transporters for smoother operations, ultimately boosting customer satisfaction.
6. Enhanced Customer Satisfaction
Satisfied customers are at the heart of a successful business. Analytics helps manufacturers align their products with customer needs:
- Monitoring Quality: Tracks production quality in real-time to ensure products meet customer standards.
- Early Issue Detection: Identifies potential quality issues before they reach the customer, reducing returns and complaints.
- Customer Feedback Analysis: Uses data from feedback and purchasing trends to tailor products.
- Market Alignment: Adjusts offerings to match changing customer preferences, fostering stronger customer relationships.
Implementation of Business Analytics
Implementing business analytics in manufacturing involves strategic steps that turn raw data into actionable insights. Each phase is critical in ensuring the data is accurate, integrated, and useful for decision-making. Here’s a breakdown of the key stages:
1. Data Collection
Data collection is the foundation of any analytics initiative. In manufacturing, data comes from various sources, such as sensors on machinery, production logs, and supply chain databases. This stage involves gathering data from:
- IoT Sensors: Real-time data from machines about performance, temperature, and operational status.
- Production Systems: Logs and records that track workflow, production speed, and output.
- Supply Chain Databases: Information on inventory levels, shipment tracking, and supplier performance.
2. Data Cleaning
Once data is collected, the next step is to clean it. This process involves removing errors, duplicates, and inconsistencies to ensure accurate and reliable data. Clean data is crucial for accurate analysis, as even minor errors can lead to misleading insights. Key aspects include:
- Error Correction: Fixing inaccuracies like misreported figures or faulty sensor readings.
- Standardization: Ensuring data follows a consistent format makes integrating and analyzing easier.
- Removing Duplicates: Eliminating redundant entries to prevent skewed analysis results.
3. Data Integration
Data integration involves combining data from multiple sources into a unified system, making it accessible for analysis. This stage often includes "business logic baking.”
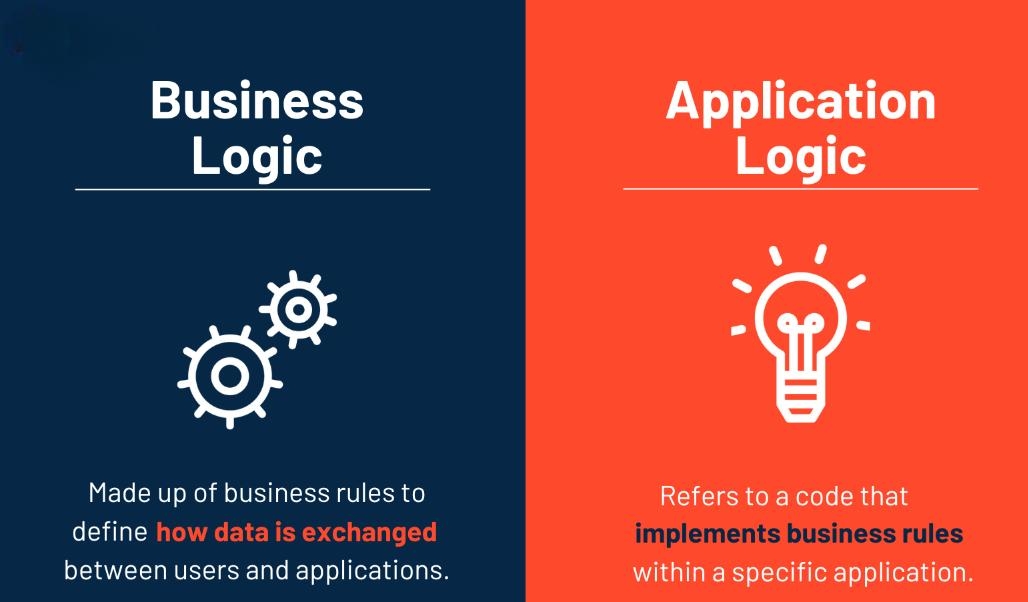
In manufacturing, "business logic baking" involves structuring data according to the specific rules and needs of the business. It means applying logic such as categorizing production data by shifts, prioritizing key suppliers, or defining conditions for equipment maintenance, ensuring that the integrated data aligns with the manufacturing company's operational goals and decision-making processes.
- Combining Data Sources: Merging data from production systems, supply chains, and customer databases.
- Business Logic: Applying rules to the data that align with business objectives, such as categorizing product types or prioritizing high-value clients.
- Data Storage: Using databases or data lakes to easily access large volumes of integrated data.
4. Data Analysis
Once the data is clean and integrated, it is ready for analysis. This step involves using statistical tools and software to derive insights for better decision-making. Key types of analysis include:
- Pattern Recognition: Identifying trends in production speed, equipment usage, or market demand.
- Anomaly Detection: Spotting irregular operations, such as sudden spikes in machine temperature.
- Trend Analysis: Understanding historical data to forecast future performance or demand.
5. Descriptive, Predictive, and Prescriptive Analytics
Manufacturing analytics leverages three types of data analysis to provide a comprehensive view of operations:
- Descriptive Analytics: Focuses on summarizing historical data to understand past performance. It answers questions like "What happened?" and helps identify patterns in production and sales data.
- Predictive Analytics: Uses historical data and machine learning models to forecast future trends. This type of analysis predicts outcomes like demand fluctuations or potential equipment failures.
- Prescriptive Analytics: Recommends specific actions based on the insights derived. It answers "What should we do?" and helps optimize decision-making by suggesting the best action, such as adjusting production schedules or changing maintenance routines.
6. Real-Time Monitoring
Real-time monitoring is a crucial component of modern manufacturing analytics, providing instant access to data from production floors and supply chains. It enables manufacturers to react quickly to changes, such as unexpected equipment downtime or shifts in demand:
- Dashboards: Display critical metrics in real-time, such as production speed, energy consumption, and inventory levels.
- Alerts and Notifications: Automatically notify managers of anomalies or equipment issues, allowing for swift corrective action.
- Continuous Feedback Loop: Real-time data allows immediate adjustments, keeping production processes efficient and minimizing disruptions.
Use Cases of Business Analytics in Manufacturing
Implementing business analytics in manufacturing can transform operations by leveraging data-driven insights. Below are some key use cases, each highlighting how analytics can address specific challenges and drive efficiency:
1. Fault Prediction and Preventive Maintenance
Real-time data analytics transforms manufacturing by enabling continuous monitoring and actionable insights. One key advancement is in fault prediction and preventive maintenance. While traditional maintenance relies on fixed schedules, real-time analytics predicts equipment failures precisely, allowing repairs to occur precisely when needed. This targeted approach minimizes repair costs, extends machine life, and minimizes production disruptions, leading to higher productivity.
2. Demand Forecasting and Inventory Management
Demand forecasting and inventory management also see significant improvements through real-time data integration. Accurate demand forecasts are crucial for maintaining balanced inventory levels, and analytics now enable manufacturers to adapt their supply chains based on live data from the production floor. This allows manufacturers to anticipate demand shifts, adjust procurement, and maintain optimal stock levels, reducing risks of overstock or shortages.

3. Price Optimization
Price optimization has become more precise through real-time insights into cycle times and production costs. With accurate data on part creation times, manufacturers can develop cost models that align prices with actual expenses, ensuring a balance between competitive pricing and profitability. This capability allows for rapid pricing adjustments in response to market shifts, helping maintain a strategic advantage.
Let's assume a Smartphone manufacturing company. The production cost of each smartphone was $300. After implementing real-time data insights, the company discovered it could produce each unit for $250. By adjusting the retail price from $600 to $550, the company sold an additional 10,000 units. This price optimization resulted in total savings of $500,000 in production costs and additional revenue of $3,000,000 from the increased sales.
4. Warranty Analysis
Warranty claims can be costly for manufacturers, but data-driven insights make managing them easier. By analyzing active warranty data, manufacturers can spot recurring product issues and make targeted design improvements, reducing future warranty costs. Additionally, warranty analysis can help identify fraudulent claims, preventing unnecessary expenses. These insights lead to better-designed products, lower support needs, and higher customer satisfaction.
5. Product Development
Product development is resource-intensive, requiring R&D investments for innovation. Traditionally, this process involved numerous physical prototypes. Data science and advanced analytics use digital twins and virtual simulations to predict product performance under real-world conditions. This reduces the need for costly trials, accelerating product launches while cutting R&D expenses.
6. Computer Vision Applications
Automated quality control has advanced significantly, shifting from basic sensors to sophisticated optical systems like thermal imaging and infrared detection. These systems feed real-time data into analytics streams, enabling precise control over production processes. This advancement boosts production speeds, lowers labor costs, and brings manufacturers closer to “lights-out” operations, where minimal human intervention is needed.
For example, in the Netherlands, Philips employs a lights-out manufacturing approach for producing electric razors, utilizing 128 robots from Adept Technology. The automated process is primarily robot-driven, with only nine human workers overseeing the final quality assurance stage of production.

7. Managing Supply Chain Risk
Data analytics extends beyond production to the entire supply chain, providing real-time visibility into materials in transit. Analyzing data from vendors and shipments allows manufacturers to adapt to disruptions quickly. This “control tower” approach enables companies to adjust production speed, order backup supplies, and activate secondary vendors as needed, ensuring a resilient supply chain.

Addressing the Key Challenges of Business Analytics in Manufacturing: Practical Solutions
Businesses often face several challenges when implementing advanced analytics. These include sourcing qualified employees with the necessary analytical skills, interpreting complex results, and integrating insights from analyses into daily decision-making.
Now, let’s explore some of the most significant challenges associated with advanced analytics:
1. Selecting the Right Data
Advanced analytics relies on vast amounts of data, and today, businesses face an overwhelming abundance of information. Selecting the right, high-quality data is crucial for ensuring the effectiveness of advanced analytics techniques. Organizations must carefully determine which data to use, identify reliable sources, and integrate it optimally.
Solution: Using incorrect or poorly sourced data or failing to integrate it properly can compromise the accuracy and usefulness of analytics results, leading to misguided decisions and costly outcomes.
2. Interpreting Data Insights
A significant challenge with advanced analytics is that business owners often need help grasping the meaning behind the data.
Solution: Understanding how various variables interact requires time and expertise, making it challenging for some individuals to utilize this information effectively in their decision-making processes.
3. Employing Skilled Personnel
Advanced analytics techniques are math-intensive and rely heavily on analytic modeling tools. Businesses need staff proficient in advanced mathematical skills, statistical methodologies, and related disciplines to uncover valuable insights. Organizations should employ data scientists alongside additional personnel who understand algorithms and AI.
However, a significant challenge is the need for more experienced data scientists. This gap still needs to be resolved, as fresh graduates will require years to gain the experience necessary to become valuable contributors. In the meantime, businesses must navigate the difficulty of needing more readily available, skilled data scientists.
Solution: Hiring dedicated staff for advanced analytics may be a significant undertaking for many small—to medium-sized enterprises. In such cases, partnering with a service provider to fulfill their business analytics needs can be a viable solution.
4. Effective Application of Advanced Analytics Techniques
Businesses must strategically apply advanced analytics techniques to derive the most valuable insights. Misapplying these methods can result in missed opportunities to gain critical information.
Solution: One practical approach is to establish internal focus groups, utilize questionnaires, or seek input from external professionals to ensure the proper techniques are employed in relevant areas.
5. Safeguarding Privacy and Security
Recent IT security breaches highlight the threats to data integrity and analytics insights. Businesses must implement stringent security measures and integrate cybersecurity protocols into their advanced analytics solutions. Furthermore, fostering a culture of security within the organization and an incident management process to address potential breaches is essential.
Solution: Companies must also prioritize respecting user and customer privacy when collecting data for analysis. In pursuit of accurate results, businesses should avoid crossing ethical lines that could compromise customer trust and safety.
6. Sharing and Collaborating
While making vast amounts of data accessible to all employees is essential, it can be challenging in practice. Accessibility, transparency, and data transfer often hinder collaboration on big data projects, especially for teams working remotely across different locations.
Solution: To address this, businesses can develop a centralized, cloud-based portal that consolidates all data, simplifying the process of sharing and collaboration among teams.
How do you select the right solution?
Manufacturing is experiencing significant transformation thanks to technologies that collect and utilize production data for improved daily decision-making.
Manufacturers should consider several key value propositions when selecting the right solution for enabling analytics on the shop floor.
Here are some essential questions to ask when evaluating analytics software:
- Can the solution gather production data in real-time?
- What are the sources of the production data? Is it collected from equipment, personnel, systems, or above?
- Is the data standardized for easy integration into out-of-the-box reports and compatibility with other production management systems (such as MES, ERP, or CMMS)?
- How quickly can the software deliver value, and what problems will it address?
We are excited to present Insia's Manufacturing Analytics Solution. Our platform facilitates real-time, automated machine data collection for precise production reporting. Stakeholders across all levels can effortlessly access ready-made reports and visualizations to minimize downtime, pinpoint production bottlenecks, enhance capacity, monitor key performance indicators (KPIs), and achieve comprehensive visibility and control on the shop floor.
Conclusion
Business analytics transforms the manufacturing industry, driving efficiency, reducing costs, and optimizing production processes. From predictive maintenance to supply chain management, the power of real-time data is clear. Manufacturers looking to stay competitive should consider implementing these data-driven solutions.
INSIA offers business analytics in the manufacturing industry by providing the following:
- Service Engineer Insights: Analyze utilization and performance across branches to improve efficiency.
- Sales and Request Tracking: Monitor sales, open service requests, and claims by customer segments, regions, and branches for better service management.
- Inventory Optimization: Track inventory and order quantities to establish minimum and maximum levels for tools and materials, ensuring optimal stock management.